AI’s carbon footprint – how does the popularity of artificial intelligence affect the climate?

With the development of artificial intelligence (AI), increasing attention is being paid not only to its potential benefits but also to the environmental consequences of its use. One key aspect of interest is AI’s carbon footprint – a measure of greenhouse gas emissions associated with the operation of AI-based systems.
In today’s world, where AI is playing an ever-increasing role in everything from medicine to transportation, entertainment to industry, understanding the environmental impact of these technologies is becoming critical. In the context of discussions about climate change and the need to reduce greenhouse gas emissions, studying the carbon footprint of AI is becoming a priority for scientists, engineers, and policymakers.
In this article, we will look at the carbon footprint of AI from different perspectives and use several popular tools, such as Midjourney and ChatGPT. We will explore how these different aspects of AI play a role in generating greenhouse gas emissions, and what factors can help reduce them. Ultimately, our goal is to explore the complexity of AI’s carbon footprint to better understand how we can use this knowledge to minimize AI’s negative impact on the planet.
Table of content
How much power does artificial intelligence AI consume?
Every time you use artificial intelligence to create an image, write a text message, or ask a chatbot a question, you are imposing a cost on the planet. In what way? For AI to work, energy is essential.
Artificial intelligence models use server infrastructure in data centers. Processing and storing data requires large amounts of energy and water for cooling. Most of this energy may come from non-renewable sources – which means emissions (burning fossil fuels to generate energy is currently the world’s largest source of greenhouse gas emissions).
The infrastructure needed to maintain all AI systems consumes as much electricity as a small country. Alex de Vries published the study in Digiconomist Joule from which he concludes that the annual demand for electricity by artificial intelligence worldwide could increase from 85.4 to 134 terawatt hours by 2027. AI’s energy demand is similar to that needed to mine cryptocurrencies. You can read about the climate impact of cryptocurrencies here.
The energy consumed by the world’s data centers accounts for 2,5% to 3,7% of global greenhouse gas emissions, more than the aviation industry. It is estimated that training a large AI model can result in the emission of approximately 300 tons of CO2.
What is the carbon footprint of AI?
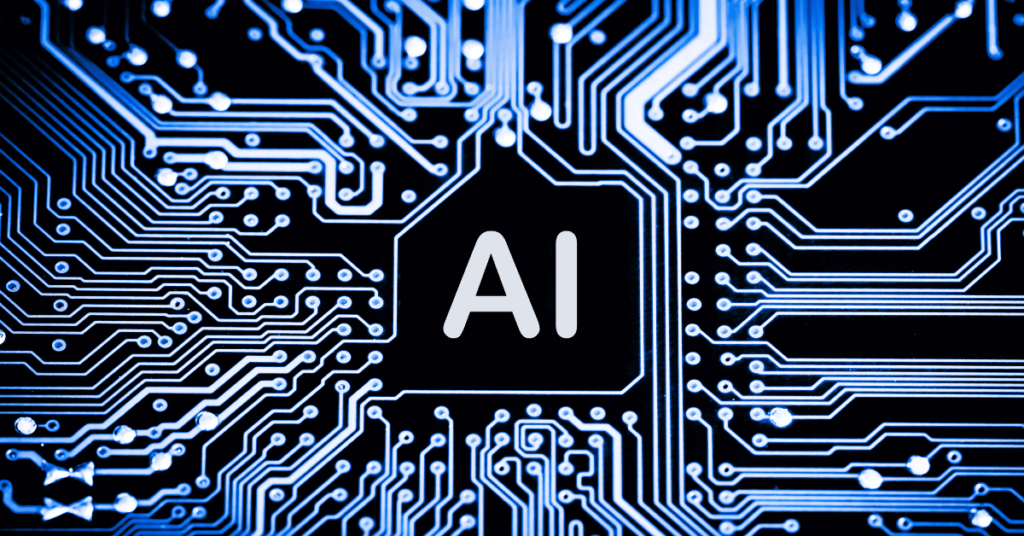
Carbon Emissions and Large Neural Network Training showed that GPT-3 training with 175 billion parameters consumed 1,287-megawatt hours of electricity and produced 502 tons of carbon dioxide emissions, which is comparable to the emissions generated by 112 gasoline-powered cars for a year.
Google, on the other hand, estimated that GPT-3’s carbon footprint is about 8.4 tons of CO2 per year.
It’s also worth keeping in mind that estimates can vary depending on several factors, including region, infrastructure provider, energy efficiency, and AI usage.
AI language model vs. carbon footprint
Large language models are not necessary for every type of task. Using smaller AI models for simpler tasks is one way to save energy – models with a narrower scope rather than those that can handle all tasks are more economical and, by using less energy, are also more environmentally friendly.
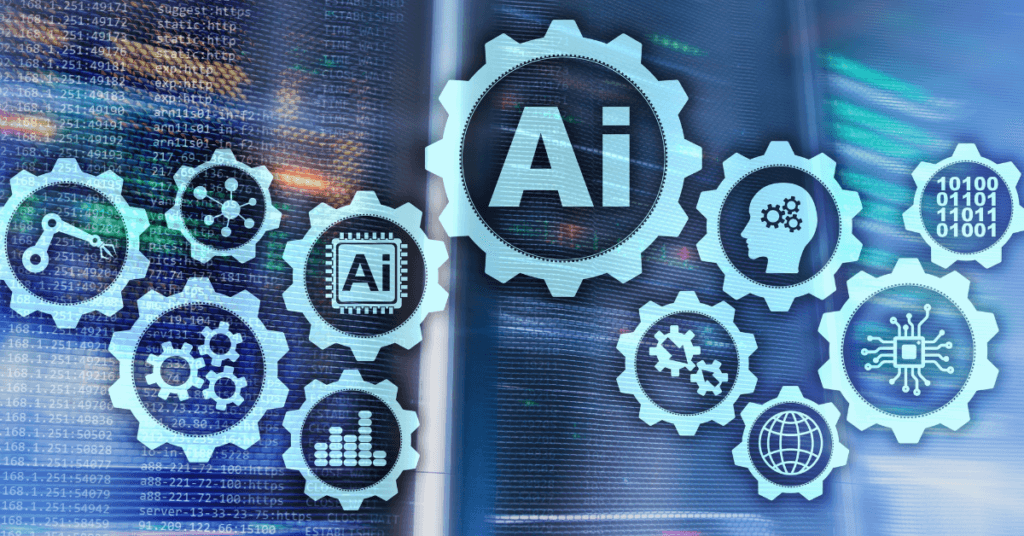
How many CO2 emissions does a ChatGPT query generate?
ChatGPT is a large and energy-intensive language model. The equivalent of each query was estimated at 4.32 g of CO2 (for comparison, a Google search is 0.2g per query). According to this calculator, 16 queries = the emissions generated by boiling a kettle. If each unique visit results in an average of 10 queries, that’s 15 trillion queries per month.
The exact environmental cost of AI activity is not known. The developers of the latest models do not provide detailed emissions figures.
Which has a greater equivalent - human article writing or AI text generation?
Although it can be difficult to determine the exact emissions of an artificial intelligence system, and different sources report different values, two key factors are critical: model training and emissions per query. Human texting generates higher emissions – the level also depends on hardware and location.
The carbon emissions of writing and illustrating are lower for AI than for humans research, published in Nature in February 2024, compares the CO2 equivalent emitted by popular text creators with human work. GPT-3 training emits about 552 metric tons of CO2e, while BLOOM training emits 50.5 metric tons of CO2e. Additional factors, such as embedded chip and server energy, as well as end-of-life recycling, have a much smaller impact compared to training and query emissions. These results indicate that the overall impact of AI queries, including both – training and operation, is typically on the order of a few grams of CO2e per query.
To calculate a writer’s carbon footprint, we took into account how fast they wrote, where they lived, and the energy consumption and emissions of the computer equipment they used to write. It also took into account whether the writer used renewable energy to power his or her computer.
What did was find? Artificial intelligence writing (via BLOOM or ChatGPT) produces 130-1500 times less CO2e per page than a human author. The results of the author comparison are shown in the graph below.
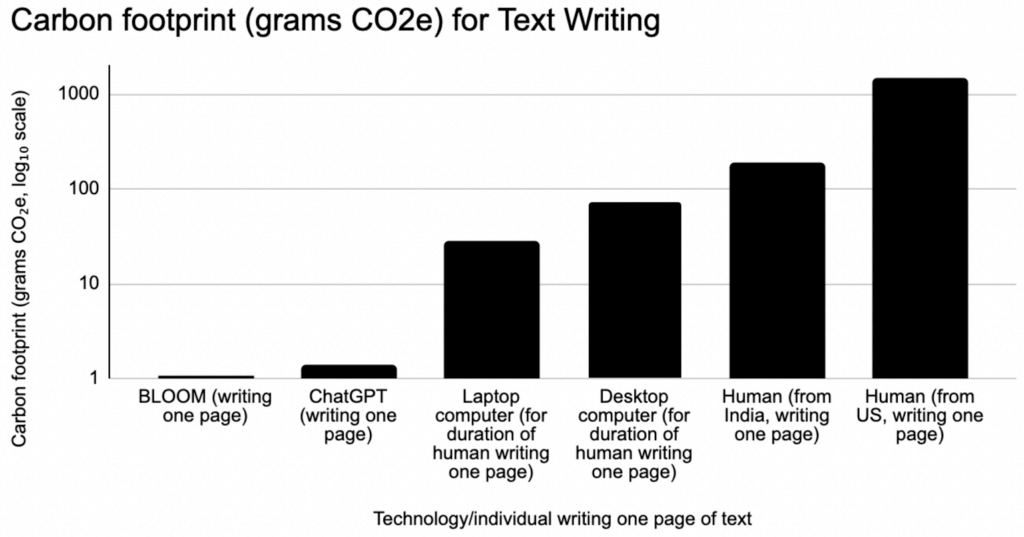
Image creation in DALL-E, Midjourney vs. human work
A study by the Hugging Face AI team and Carnegie Mellon University found that image generation is the most energy-intensive AI-based task and that generating 1,000 images using a powerful model like Stable Diffusion XL generates carbon emissions equivalent to driving 4.1 miles in a gasoline-powered car.
Nature, on the other hand, in the study cited in the paragraph above, compares the human work of image generation to that of Midjourney and DALL-E. In examining two well-known AI image generation engines, DALL-E2 and Midjourney, it was found that DALL-E2 is based on GPT-3, as is ChatGPT. The carbon footprint of DALL-E2 is estimated to be similar to that of ChatGPT, at 2.2 g CO2e per query.
As for Midjourney, CEO David Holz says that image generation requires about 1,000 trillion operations, suggesting a carbon footprint of about 1.9 g CO2e using Nvidia A100 GPUs, which consume 400 W of power.

Image creation by artificial intelligence (via DALL-E2 or Midjourney) emits 310 to 2,900 times less CO2e per image than the human creation process. The use of artificial intelligence generates significantly less CO2e than using a computer to assist humans in image creation.
Water footprint of AI
In a conversation about emissions, it’s impossible not to mention ChatGPT’s water footprint. According to a study by the University of California, Riverside, a conversation of about 20 queries uses half a liter of water, the equivalent of a small plastic bottle. ChatGPT training can use as much water as producing 370 BMWs or 320 Teslas.
Shaolei Ren, an associate professor of electrical and computer engineering at the University of California, Riverside, wrote in his study from 2023:
It takes about 2,200 gallons of ultrapure water (UPW) to produce a microprocessor. Putting that aside, training a large language model such as GPT-3 can consume millions of gallons of fresh water, and running a GPT-3 inference for 10-50 queries uses 500 milliliters of water, depending on when and where the model is hosted. GPT-4, the model currently used by ChatGPT, is said to be much larger in size, and therefore likely to consume more water than GPT-3.
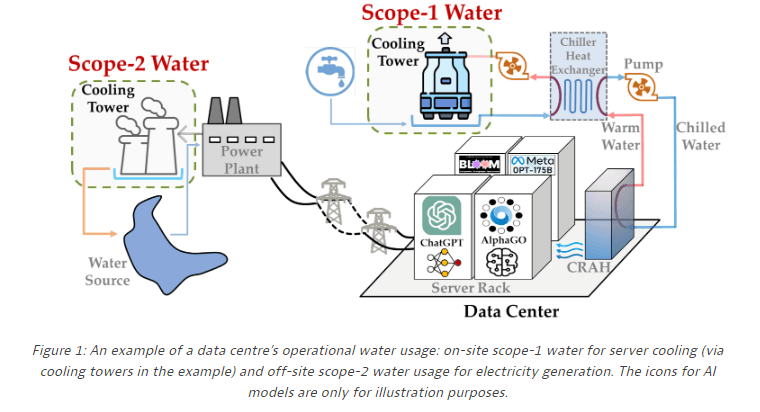
The water used to prevent overheating in data centers is usually evaporated, which means it cannot be reused.
Can AI be greener?
Yes, there are ways to make artificial intelligence (AI) greener:
- Optimize algorithms: Optimizing algorithms can reduce the resources and energy needed to train and run AI models.
- Sustainable energy sources: Moving data centers to sustainable energy sources, such as solar or wind power, can reduce the environmental impact of AI.
- Recycling and reuse: Promoting recycling and reuse practices for hardware used in AI processing can reduce the negative environmental impact.
- Improved cooling: Using more efficient cooling technologies in data centers can reduce the amount of energy needed to maintain appropriate temperatures. Microsoft is testing an underwater data center system.
- Applying AI to conservation: Using AI to optimize environmental processes, such as monitoring climate change, managing natural resources, or protecting biodiversity, can help create more sustainable practices.
How to use artificial intelligence for the good of the Earth?
Artificial intelligence has great potential to help protect the environment and create a more sustainable future for our planet. However, it is also important to manage this technology properly to ensure that its impact is positive for the environment and society as a whole.
AI makes it possible to sift through large data sets, such as satellite images, to help scientists monitor climate change. It can also be used to build better model climate patterns, identify trends, and predict future changes, contributing to a better understanding and more effective mitigation of climate change. Other applications include water conservation, firefighting, and the identification and recovery of recyclable materials.
Scientists of the National Oceanic and Atmospheric Administration are using AI to improve climate models, weather forecasts, and other Earth-related applications and systems. The United Nations Environment Programme is using AI to analyze atmospheric carbon dioxide concentrations, changes in glacier mass, and sea level rise.
Summary
AI consumes significant amounts of natural resources, and its carbon footprint can be significant, especially when training large language models. However, it is also important to recognize the positive impact that AI can have on organizing and protecting the environment. AI supports monitoring climate change, analyzing ecosystems, optimizing energy consumption, and many other activities that help protect the planet.
The key challenge seems to be striking a balance between the user benefits of AI technology and minimizing its negative impact on the environment. By developing more efficient algorithms, using energy resources more consciously, and promoting sustainable practices in the technology sector, we can make progress in both artificial intelligence and environmental protection.
In conclusion, while artificial intelligence is not without its environmental challenges, the right approach and innovative solutions can help harness its potential in a way that supports our efforts to be sustainable and protect the planet for future generations.